报告题目:Graph model for High dimensional data classification
报告人:Xue-Cheng Tai, Department of Mathematics, Hong Kong Baptist University.
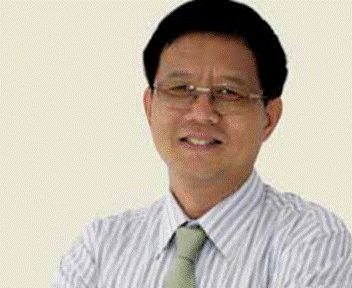
邀请人:袁景
报告时间:2021年11月24日(周三)15:00-16:00
报告地点:腾讯会议:143 252 332
报告人简介:Xue-Cheng Tai教授是香港浸会大学数学系主任,挪威卑尔根大学数学系教授,长期从事计算数学、图像计算及反问题,变分优化算法及应用,对水平集的变分优化算法、区域分割和多重网格的变分计算,以及大规模优化计算等计算数学基础问题作出突出贡献,共发表各类学术期刊文章超过210篇,以及编辑多本学术专著。主持组织各类专业国际学术会议,包括SSVM、EMMCVPR等,同时担任多个顶级专业期刊,如SIAM J. Imaging Science,Journal of Scientific Computing等编委成员。1993年获德国洪堡学者;2009年,由于在科学计算方面的突出贡献,获得第8届“冯康奖”;2011年,获得新加坡南洋理工大学“南洋突出研究贡献奖”。
报告摘要:This talk contains 3 parts.
1. In the 1st part, we present our work to use graph models for high dimensional data classification. Especially, we show how to get fast algorithms using min-cut and max-flow algorithms. Moreover, we add a regional force to our model which has demonstrated to give superior accuracy for many applications.
2. In the second part, show that the well-know modularity maximization algorithm is in fact is volume balancing model. Using total variation on graphs, we show that we can turn the modularity maximization into a minimization problem with volume balancing property with a convex energy functional. This is a new observation and also gives some new ways to solve the modularity minimization problems.
3. In the 3rd part, we will use similar ideas to add spatial regularization effect to popular deep neural networks. We use numerical experiments to show that the regularized DNN always has smooth boundary when used for image segmentation and similar classification problems.