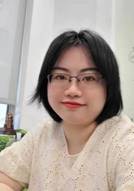
报告题目
A Novel Surrogate-Function-Based Paradigm for Large-Scale Convex Composite Optimization
报告摘要
In this talk, I will introduce a novel paradigm called the Approximate Method of Multipliers (AMM) for solving a generic, large-scale convex composite optimization problem. AMM attempts to approximate the classic Method of Multipliers by virtue of a surrogate function with numerous options. It can be specialized to different types of new algorithms (e.g., proximal, second-order, gradient-tracking, etc.), and generalizes a broad span of existing first-order and second-order methods that were originally developed via different rationales. In contrast to the earlier unifying optimization frameworks, which can barely reduce to second-order algorithms and require separable surrogate functions for distributed problem solving, AMM can effortlessly include second-order information through its surrogate function, and enables distributed implementation with both separable and non-separable surrogate function forms designed via ideas like Bregman divergence and convex conjugate functions. AMM is able to achieve an O(1/k) rate of convergence to optimality, and the convergence rate becomes linear when the problem is locally restricted strongly
convex and smooth. Such convergence rates provide new or stronger convergence results to many prior methods that can be viewed as specializations of AMM.
个人简介
陆疌,女,上海科技大学信息科学与技术学院常任副教授,2007年本科毕业于上海交通大学信息工程系,2011年获美国俄克拉荷马州立大学电子与计算机工程博士学位。2012年至2015年,她分别在瑞典皇家理工学院和瑞典查尔姆斯理工大学做博士后研究员,并于2015年加入上海科技大学。她的主要研究方向为分布式优化、优化理论与算法、网络动力系统。
报告时间
2022年11月29日,15:00~18:00
报告地点
腾讯会议:744-831-861